Proposed by Burkhard and Keller in 1973, the BK-Tree is a data structure used for spell checking based on the Levenshtein Distance between two words, which is basically the number of changes you need to make to a word to turn it into another word. Some example distances:
LevenshteinDistance(cook, book) -> 1
LevenshteinDistance(cook, books) -> 2
LevenshteinDistance(what, water) -> 3
To make the last example clear, the distance between what and water is based on three moves, the first is to drop the h, then add the e and finally add the r. You can use this distance to work out how close someone is to spelling a word correctly, for instance if I want to know out of the set of words [cook, book, books, what, water] which word the misspelling wat is closest to I could do a full scan of the list and find the words with the lowest distance:
LevenshteinDistance(wat, cook) -> 4
LevenshteinDistance(wat, book) -> 4
LevenshteinDistance(wat, books) -> 5
LevenshteinDistance(wat, what) -> 1
LevenshteinDistance(wat, water) -> 2
Based on that search I can determine the user probably meant the word what due to it having the lowest distance of 1.
This works in the case of a small number of words since O(n) isn’t bad in this case, however if I wanted to scan a full dictionary for the closest match O(n) isn’t optimal. This is where a BK-Tree comes in.
To build a BK-Tree all you have to do is take any word from your set and plop it in as your root node, and then add words to the tree based on their distance to the root. For instance if I started a tree with the word set [book, books, cake] then my tree would look like this if I started by making the word book my root node:
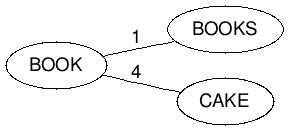
This is because:
LevenshteinDistance(book, books) -> 1
LevenshteinDistance(book, cake) -> 4
Of course now if we add the word boo to the mix we get a conflict because:
LevenshteinDistance(book, boo) -> 1
which collides with books. To handle this we now have to chain boo from books by making it a child of books based on their Levenshtein Distance.
LevenshteinDistance(books, boo) -> 2
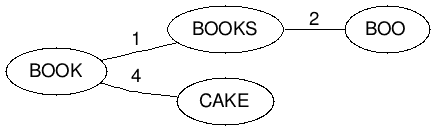
We continue to use this strategy as we add words, so if we throw in [Cape, Boon, Cook, Cart] then we get this:
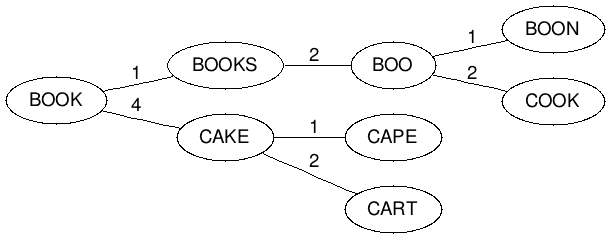
Now that we have our structure the obvious question is how do we search it? This is simple as now all we need to do is take our misspelled word and find matches within a certain level of tolerance, which we’ll call N. We do this by taking the Levenshtein Distance of our word and compare it to the root, then crawl all nodes that are that distance ±N.
For example, if we searched for the closest match to the misspelling caqe within the tolerance of 1 in the above structure we would crawl it like this:
1) LevenshteinDistance(caqe, book) -> 4
a) Check (4 <= 1) - Don't add book as a match
b) Crawl all edges between 3 and 5 (4-1,4+1)
2) Crawl into edge path 4 from book
3) LevenshteinDistance(caqe, cake) -> 1
a) Check (1 <= 1) - Add cake as a match
b) Crawl all edges between 0 and 2 (1-1, 1+1)
4) Crawl into edge path 1 from cake
5) LevenshteinDistance(caqe, cape) -> 1
a) (1 <= 1) - Add cape as a match
6) Crawl into edge path 2 from cake
7) LevenshteinDistance(caqe, cart) -> 2
a) Check (2 <= 1) - Don't add cart as a match
From this example it appears we can now find misspellings at a O(log n) time, which is better than our O(n) from before. This however doesn’t tell the whole story, because our tolerance can drastically increase the number of nodes we need to visit in order to do the search. A discussion over a BK-Tree implementation in Lucene seems to indicate a tolerance of 2 should limit a dictionary search to 5-10% of a tree, while someone else did some tests with random strings to get their own metrics (though random strings are a poor representation of real-world misspellings). In practice the speed improvement of this structure is going to be highly dependent on the set of words you are using and the typical inputs you run into so independent research is needed to see if it applies to any specific problem (such as matching misspelled city names).
As always here’s a C# implementation under the MIT license:
/*
The MIT License (MIT)
Copyright (c) 2013
Permission is hereby granted, free of charge, to any person obtaining a copy of this software and associated documentation files (the "Software"),
to deal in the Software without restriction, including without limitation the rights to use, copy, modify, merge, publish, distribute, sublicense,
and/or sell copies of the Software, and to permit persons to whom the Software is furnished to do so, subject to the following conditions:
The above copyright notice and this permission notice shall be included in all copies or substantial portions of the Software.
THE SOFTWARE IS PROVIDED "AS IS", WITHOUT WARRANTY OF ANY KIND, EXPRESS OR IMPLIED, INCLUDING BUT NOT LIMITED TO THE WARRANTIES OF MERCHANTABILITY,
FITNESS FOR A PARTICULAR PURPOSE AND NONINFRINGEMENT. IN NO EVENT SHALL THE AUTHORS OR COPYRIGHT HOLDERS BE LIABLE FOR ANY CLAIM, DAMAGES OR OTHER LIABILITY,
WHETHER IN AN ACTION OF CONTRACT, TORT OR OTHERWISE, ARISING FROM, OUT OF OR IN CONNECTION WITH THE SOFTWARE OR THE USE OR OTHER DEALINGS IN THE SOFTWARE.
*/
using System;
using System.Collections;
using System.Collections.Generic;
using System.Collections.Specialized;
using System.Linq;
public class BKTree
{
private Node _Root;
public void Add(string word)
{
word = word.ToLower();
if (_Root == null)
{
_Root = new Node(word);
return;
}
var curNode = _Root;
var dist = LevenshteinDistance(curNode.Word, word);
while (curNode.ContainsKey(dist))
{
if (dist == 0) return;
curNode = curNode[dist];
dist = LevenshteinDistance(curNode.Word, word);
}
curNode.AddChild(dist,word);
}
public List<string> Search(string word, int d)
{
var rtn = new List<string>();
word = word.ToLower();
RecursiveSearch(_Root, rtn, word, d);
return rtn;
}
private void RecursiveSearch(Node node, List<string> rtn, string word, int d )
{
var curDist = LevenshteinDistance(node.Word, word);
var minDist = curDist - d;
var maxDist = curDist + d;
if (curDist <= d)
rtn.Add(node.Word);
foreach (var key in node.Keys.Cast<int>().Where(key => minDist <= key && key <= maxDist))
{
RecursiveSearch(node[key], rtn, word, d);
}
}
public static int LevenshteinDistance(string first, string second)
{
if (first.Length == 0) return second.Length;
if (second.Length == 0) return first.Length;
var lenFirst = first.Length;
var lenSecond = second.Length;
var d = new int[lenFirst + 1, lenSecond + 1];
for (var i = 0; i <= lenFirst; i++)
d[i, 0] = i;
for (var i = 0; i <= lenSecond; i++)
d[0, i] = i;
for (var i = 1; i <= lenFirst; i++)
{
for (var j = 1; j <= lenSecond; j++)
{
var match = (first[i - 1] == second[j - 1]) ? 0 : 1;
d[i, j] = Math.Min(Math.Min(d[i - 1, j] + 1, d[i, j - 1] + 1), d[i - 1, j - 1] + match);
}
}
return d[lenFirst, lenSecond];
}
}
public class Node
{
public string Word { get; set; }
public HybridDictionary Children { get; set; }
public Node() { }
public Node(string word)
{
this.Word = word.ToLower();
}
public Node this[int key]
{
get { return (Node)Children[key]; }
}
public ICollection Keys
{
get
{
if(Children == null)
return new ArrayList();
return Children.Keys;
}
}
public bool ContainsKey(int key)
{
return Children != null && Children.Contains(key);
}
public void AddChild(int key, string word)
{
if(this.Children == null)
Children = new HybridDictionary();
this.Children[key] = new Node(word);
}
}